THE LATEST
AI speeds up discovery of energy, quantum materials
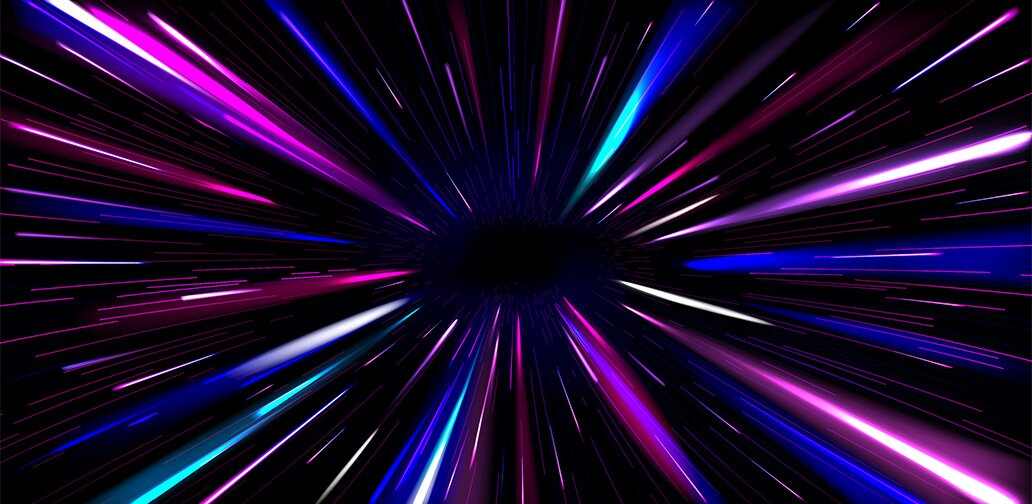
In a landmark collaboration, Tohoku University in Japan and the esteemed Massachusetts Institute of Technology (MIT) have unveiled an advanced AI tool. This tool is poised to revolutionize the discovery of energy and quantum materials, a development that is set to reshape the landscape of optoelectronic device development and drive scientific innovation to unprecedented heights.
Under the leadership of Nguyen Tuan Hung from Tohoku University's Frontier Institute for Interdisciplinary Science and Mingda Li from MIT's Department of Nuclear Science and Engineering, a research team has introduced an AI model. This model accelerates the calculation of high-quality optical spectra with unparalleled speed and precision. The model's ability to match the accuracy of quantum simulations while operating a million times faster opens up vast potential for accelerating the development of photovoltaic and quantum materials, with significant implications for the energy and semiconductor industries.
Understanding the optical properties of materials is a key factor in advancing optoelectronic devices. These devices, such as LEDs, solar cells, photodetectors, and photonic integrated circuits, are instrumental in driving innovation in the semiconductor industry. Traditionally, performing calculations based on the fundamental laws of physics required complex computations and immense computational resources, posing challenges in swiftly evaluating numerous materials. The AI model introduced by the research team overcomes this hurdle, promising to discover novel photovoltaic materials for efficient energy conversion and gain profound insights into the underlying physics of materials through their optical spectra.
Nguyen Tuan Hung, the lead author of the groundbreaking study, expressed his fascination with optics in condensed matter physics, noting how the AI model adeptly grasps sophisticated physics concepts through the Kramers-Krönig relation. This innovative approach eliminates the limitations posed by experimental laser wavelengths and the complexity of simulations, presenting a more efficient method for predicting the optical spectra of diverse materials.
The introduction of graph neural networks (GNNs) to predict material properties represents a significant step forward in machine learning. However, the challenge of achieving universality in the representation of crystal structures prompted the researchers to devise a novel universal ensemble embedding, unifying multiple models or algorithms to enhance prediction accuracy without altering neural network structures.
This universal layer, seamlessly integrated into any neural network model, heralds a new era of precision in optical predictions based solely on crystal structures, with wide-ranging applications in material screening for high-performance solar cells and identifying quantum materials on the horizon. Additionally, the research team aims to expand their databases to include other material properties, such as mechanical and magnetic characteristics, further enhancing the AI model's predictive capabilities based on crystal structures.
As we witness this remarkable fusion of cutting-edge technology and visionary scientific exploration, we are reminded of the endless possibilities of collaboration and innovation. The pioneering work of the research team serves as a beacon of inspiration, illuminating a path toward a future where AI-driven discoveries propel us closer to unlocking the transformative potential of energy and quantum materials. For more information, refer to the research publication in Advanced Materials titled "Universal Ensemble-Embedding Graph Neural Network for Direct Prediction of Optical Spectra from Crystal Structures." Contact Nguyen Tuan Hung at nguyen.tuan.hung.e4@tohoku.ac.jp for further insights into this monumental research endeavor.
