BIOLOGY
Beer's program should shed light on complex diseases
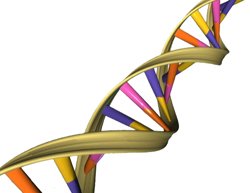
Up to one-fifth of human DNA act as dimmer switches for nearby genes, but scientists have long been unable to identify precisely which mutations in these genetic control regions really matter in causing common diseases. Now, a decade of work at Johns Hopkins has yielded a supercomputer formula that predicts with far more accuracy than current methods which mutations are likely to have the largest effect on the activity of the dimmer switches, suggesting new targets for diagnosis and treatment of many diseases.
A summary of the research will bepublished online June 15 in the journal Nature Genetics.
“Our computer program can comb through the genetic information from a specific cell type and predict which ‘dimmer switch’ mutations are most likely to alter the cell’s gene activity, and therefore its function,” says Michael Beer, Ph.D., associate professor of biomedical engineering at the Johns Hopkins University School of Medicine.
“The plan is to continually improve the formula as we learn more about these regulatory regions,” says Beer, “but already it can narrow down a list of disease-associated mutations by a factor of 20, allowing researchers to focus on the ones that are most likely to matter.”
Researchers around the world have sequenced the genomes of many patients suffering from common multigene diseases, looking for shared mutations in their control regions. The trouble is, Beer says, that these studies yield hundreds of mutations, most of which are benign. So he and his team of researchers set out to design a computer program that could learn the difference between mutations that are likely to affect gene activity levels and those that likely won't.
“There are a lot of common diseases, like diabetes, that are probably the result of several different mutations in control regions. The mutations don't directly cause a change in the proteins made, but they impact their abundance,” he says, and sorting out which ones matter most in diseases is key to advancing treatments.
The task has been difficult, Beer says, because not all mutations are created equal. A single alteration, say from a cysteine (C) to a guanine (G) in the four-letter alphabet of DNA, will have drastically different effects based on where it occurs in the genome, he explains.
“If it occurs in the middle of a gene that encodes a crucial protein, it could alter the code in such a way that no protein is made and the organism dies, or it could have no effect whatsoever if the function of the protein isn’t altered by the change,” he says. The same extremes could be true if the C to G mutation occurred outside of a gene, in a control region: The mutation could cause the region to stop working altogether, or it could have no effect. And between those extremes is everything else.
To develop the new formula, Beer says his team first “trained” its computer program to recognize potential control regions using a property called DNase sensitivity. DNase is an enzyme that cuts DNA wherever it is not tightly wound. The openness of particular sequences of DNA varies among different types of cells, and only control regions in open DNA can be active. How vulnerable certain stretches of DNA are to DNAse is therefore an indication of which control regions are important in a given cell type, Beer says.
Dongwon Lee, Ph.D., then a graduate student in Beer’s laboratory, taught the computer program to recognize the features of DNase-sensitive sequences in a type of cancer cell by giving the computer a list of already known sequences. It then predicted the rest of the DNase-sensitive sequences and measured how much individual sections of a sequence contributed to that region’s overall DNase sensitivity.
The computer then simulated “mutating” every DNA letter in turn and recalculated each section’s contribution to DNase sensitivity. The larger the change in sensitivity after a given mutation, the more likely it is that that mutation will affect gene activity levels in the cell, Beer says.
To test the validity of the formula, the team compared their computer predictions to the predictions made by alternative programs. When the programs’ “rules” were set to be equally thorough in their searches, Beer’s program was 56 percent accurate — 10 times more accurate than the next best program.
To further directly test the formula, Beer worked with Andrew McCallion, Ph.D., an associate professor at the McKusick-Nathans Institute of Genetic Medicine at the Johns Hopkins University School of Medicine, to predict the impact of mutations in the control regions for two pigment-related genes in mouse melanocytes (skin pigment cells). They then selected 40 mutations with different levels of predicted impact and tested their effect in melanocytes grown in the laboratory. When they measured the activity levels of the two genes, they found that there was a strong correlation between the program’s prediction and the actual change experienced by the cells.
“My group has been working for over a decade to shed some light on the nature of regulatory mutations in common disease,” McCallion says. “The synergy of our careers and our strategies bring the Beer group and mine to an exciting place in this effort. By training the computer program with the right cellular material, we can now predict the consequences of previously undecipherable regulatory sequence mutations.”
Beer and his team repeated this targeted testing of their formula in mouse and human liver cells and in human leukemia cells, with similar results. They also tested their formula on three control region mutations already known to affect cholesterol levels, hemoglobin levels and prostate cancer. Again they found that these mutations drew higher computer scores than other mutations in the same control regions.
Finally, the team examined the control regions for T helper cells, a type of immune cell that can contribute to autoimmune diseases when its genes become disregulated. Their calculations identified 15 different control region mutations associated with nine different immune system disorders, from allergies to multiple sclerosis and Crohn’s disease. Importantly, Beer says, previous studies had associated nine of the same control regions with immune disorders, but they had not been able to hone in on the exact mutation that mattered.
Beer says: “The next step is to collect cells from patients with these autoimmune diseases, test their gene activity levels and find out if our predictions were right. If so, it should help us determine how the activity is being perturbed and how we can fix it.” The same process can theoretically be repeated on many other diseases, providing timesaving insights for each.
Other authors of the report include David Gorkin, Maggie Baker, Benjamin Strober and Alessandro Asoni of the Johns Hopkins University School of Medicine.