ECONOMICS
Promises & pitfalls: Machine learning in financial markets
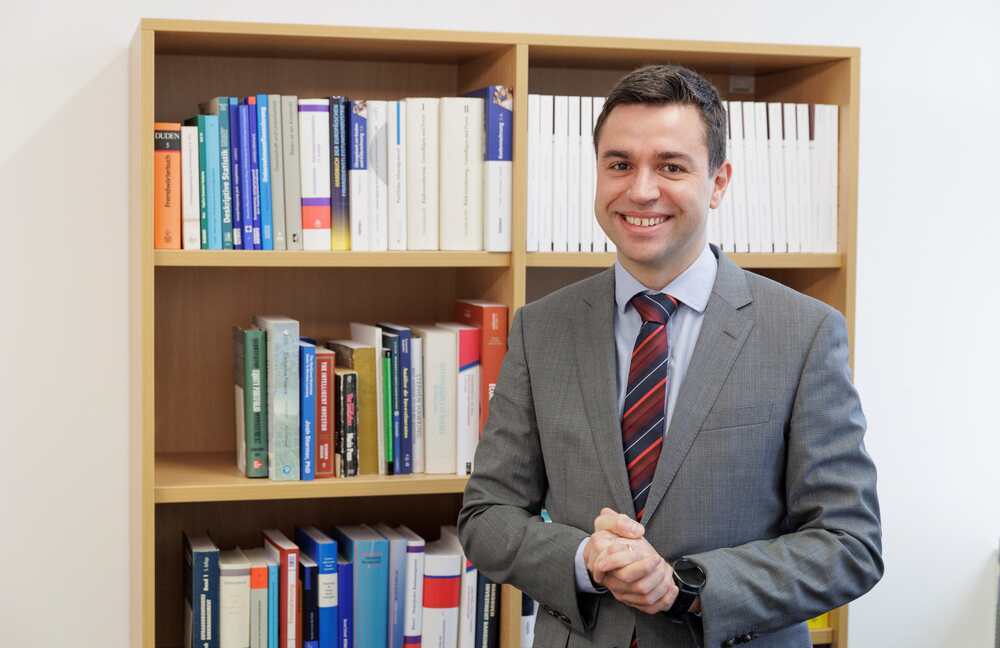
The application of machine learning (ML) in the financial market has been touted as a game-changer for stock return predictions. A recent study conducted by researchers from Kaiserslautern and Munich, published in the "Journal of Asset Management," explores the potential of ML methods to enhance stock return forecasting. While the findings showcase remarkable accuracy and improved returns, experts urge caution, highlighting the need for a skeptical evaluation of this emerging technology.
ML methods, a branch of artificial intelligence (AI), offer the promise of aggregating numerous factors and market anomalies to improve stock return predictions. Traditional methods often fall short, particularly in global stock investments. The researchers embarked on a quest to determine if ML could provide a solution, and their study presents fascinating insights.
Professor Dr. Vitor Azevedo from the University Kaiserslautern-Landau, co-author of the study, explains the significance of capital market anomalies in stock forecasts. Over 400 of these phenomena, identified by renowned financial journals, have been deemed predictive of stock returns. For instance, the well-known "Price-Earnings Ratio" (PER) and the "Short-Term Reversal" effect have shown potential in guiding investment decisions. However, understanding which anomalies are relevant, how they interact, and their combined impact poses a complex challenge.
In this undertaking, the researchers examined various ML approaches and analyzed a staggering 1.9 billion stock-month-anomaly observations spanning nearly four decades across 68 countries. Their findings are impressive, suggesting that ML models outperform traditional methods. Predicting stock returns with astonishing accuracy, these ML models recorded an average monthly return of up to 2.71 percent, far surpassing the meager 1 percent achieved by traditional approaches.
The implications of this research are tantalizing, offering financial managers the prospect of developing new stock price models and potentially increasing profitability. However, experts caution that there are several factors that demand careful consideration.
One critical aspect lies in the preparation of data, emphasizing the importance of correctly incorporating outliers and missing values, especially when working with international data. Ethical and regulatory concerns are also raised, as the deployment of AI techniques in financial markets warrants extensive review.
While these findings highlight the potential of ML in the financial sector, skepticism is vital. The complexity of stock predictions and the volatile nature of financial markets mean that applying ML methods alone may oversimplify a multifaceted problem. Critics argue that there is a risk of relying too heavily on machine learning algorithms and overlooking crucial factors that cannot be captured by computational models alone, such as economic trends, geopolitical events, and human behavior.
It is crucial to strike a balance between technological innovation and traditional financial expertise. Combining ML methods with the experience and insights of financial analysts can create a stronger foundation for informed decision-making. Additionally, stakeholders must remain vigilant regarding potential biases embedded in ML algorithms and transparency in their implementation.
The study, titled "Stock market anomalies and machine learning across the globe," urges the financial industry to approach the integration of ML cautiously. While its potential cannot be ignored, rigorous evaluation, validation, and an ongoing dialogue among experts are vital to harness the benefits of this emerging technology while mitigating its inherent risks.