ENGINEERING
Machine teaching holds the power to illuminate human learning
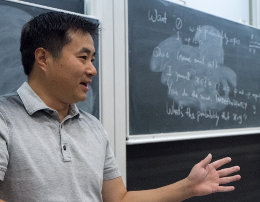
Human learning is a complex, sometimes mysterious process. Most of us have had experiences where we have struggled to learn something new, but also times when we've picked something up nearly effortlessly.
What if a fusion of computer science and psychology could help us understand more about how people learn, making it possible to design ideal lessons?
That long-range goal is moving toward reality thanks to an effort led by professors in the University of Wisconsin-Madison departments of computer sciences, psychology and educational psychology. Their collaborative research aims to break new ground in what computer scientist Jerry Zhucalls "machine teaching" — a twist on the more familiar concept of machine learning.
"My hope is that machine teaching has an impact on the educational world. It's quite different from how people usually think about education," says Zhu. "It will give us optimal, personalized lessons for real, human students."
Machine learning is a well-established subfield of computer science in which experts develop mathematical tools to help computers learn from data and detect patterns. The machine learner (the computer) is like a student. The goal of machine learning is to develop models that will prove useful in the future when dealing with large, often unwieldly data sets. Practical tasks like speech recognition are aided by machine learning.
Machine teaching turns this concept on its ear. Rather than dealing with pools of data and not knowing at the outset what patterns might be revealed through analysis, the researcher in a machine teaching arrangement already knows what knowledge he or she wants to impress upon the learner.
Timothy Rogers
Machine teaching uses sophisticated mathematics to allow researchers to model actual human students and devise the best possible lessons for teaching them. While the definition of "best" in a particular setting is up to the teacher, one example could be identifying the smallest number of exercises needed for a particular student to grasp a concept. Or, as Zhu puts it, "Can five really good questions teach the material, rather than 20?"
While this work is still in its early stages, it has immense potential to impact education.
Timothy T. Rogers, a professor of cognitive psychology at UW-Madison and one of Zhu's collaborators, explains how computer science and psychology come together.
"In order for the machine teaching approach to work, it needs a good model of how the learner behaves — that is, how the learner's behavior changes with different kinds of learning or practice experiences," Rogers says. "Also, the model needs to be computational; it has to be able to make concrete, quantitative predictions about the learner's behavior."
"Ultimately, we hope that the work can be used to help teachers develop lesson plans and curricula that promote learning in a wide variety of fields," Rogers says, citing math, science and reading as examples. "And, just as important, the effort to bring cognitive models of learning to bear on real-world problems is bound to lead to important new advances in our understanding of how people learn generally."
“It's quite different from how people usually think about education. It will give us optimal, personalized lessons for real, human students.”
Jerry Zhu
Zhu presented some of his research earlier this year in Austin, Texas, at the 29th annualConference on Artificial Intelligence, organized by the Association for the Advancement of Artificial Intelligence.
A two-year seed grant from the UW-Madison Graduate Schoolcurrently supports this work. Future funding from outside sources will be sought.
"With machine teaching, it's conceptually easy, but quite challenging to implement in the real world. It's a major undertaking," says Zhu.
In addition to Zhu and Rogers, the UW research team includes computer sciences professors Michael Ferris, Bilge Mutlu andStephen Wright; engineering professor Rob Nowak; psychology professor Martha Alibali; and educational psychology professorsMartina Rau and Percival Matthews.
Machine teaching probes fundamental mathematical and scientific concepts. In part because of that, the team's research is open-ended at this stage.
